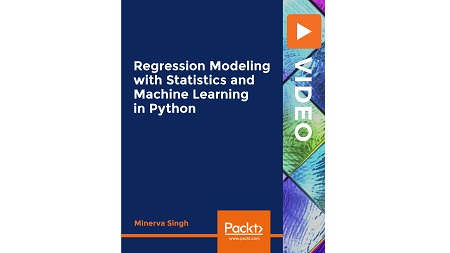
English | MP4 | AVC 1280×720 | AAC 44KHz 2ch | 6h 08m | 811 MB
This hands-on, regression-analysis bootcamp will help you master practical statistical modeling and machine learning in Python
This course is your one-shot guide to statistical and machine learning analysis. This course will teach you regression analysis (for both statistical data analysis and machine learning) in Python—all in a practical, hands-on way.
Specifically, the course will:
- Take you from a basic level of statistical knowledge to a level where you can perform some of the most common advanced regression analysis-based techniques
- Equip you to use Python to perform various statistical and machine learning data analysis tasks
- Introduce you in a hands-on way to some of the most important statistical and machine learning concepts, so you can apply them to practical data analysis and interpretation
You will get a strong background in some of the most important statistical and machine learning concepts and their applications in regression analysis. You will be able to decide which regression analysis techniques are best suited for answering your research questions and most applicable to your data; then you’ll interpret the results.
This is a practical, hands-on course—we spend time dealing with some theoretical concepts related to both statistical and machine learning regression analysis.
Learn
- Harness the power of Anaconda/iPython for practical data science
- Read in data into the Python environment from different sources
- Implement classical statistical regression modeling techniques such as linear regression in Python
- Implement machine learning-based regression modeling techniques such as Random Forests and kNN for predictive modeling
- Master neural network- and deep learning-based regression
Table of Contents
INTRODUCTION TO THE COURSE – The Key Concepts and Software Tools
1 Welcome to the Course
2 Python Data Science Environment
3 For Mac Users
4 IPython in Browser
5 Python Data Science Packages to Be Used
Read in Data from Different Sources with Pandas
6 What are Pandas
7 Read in Data from CSV
8 Read in Excel Data
9 Read in HTML Data
Data Cleaning & Munging
10 Remove Missing Values
11 Conditional Data Selection
12 Data Grouping
13 Data Subsetting
14 Ranking & Sorting
15 Concatenate
16 Merging & Joining Data Frames
Statistical Data Analysis-Basic
17 What is Statistical Data Analysis
18 Some Pointers on Collecting Data for Statistical Studies
19 Explore the Quantitative Data – Descriptive Statistics
20 Grouping & Summarizing Data by Categories
21 Visualize Descriptive Statistics-Boxplots
22 Common Terms Relating to Descriptive Statistics
23 Data Distribution- Normal Distribution
24 Check for Normal Distribution
25 Standard Normal Distribution and Z-scores
26 Confidence Interval-Theory
27 Confidence Interval-Calculation
Regression Modelling for Defining Relationship between Variables
28 Explore the Relationship Between Two Quantitative Variables
29 Correlation Analysis
30 Linear Regression-Theory
31 Linear Regression-Implementation in Python
32 Conditions of Linear Regression
33 Conditions of Linear Regression-Check in Python
34 Polynomial Regression
35 GLM – Generalized Linear Model
36 Logistic Regression
Machine Learning for Data Science
37 How is Machine Learning Different from Statistical Data Analysis
38 What is Machine Learning (ML) About Some Theoretical Pointers
Machine Learning Based Regression Modelling
39 What Is This Section About
40 Data Preparation for Supervised Learning
41 Pointers on Evaluating the Accuracy of Classification and Regression Modelling
42 RF-Regression
43 Support Vector Regression
44 knn-Regression
45 Gradient Boosting-regression
46 Theory Behind ANN and DNN
47 Regression with MLP
Miscellaneous Information
48 Using Colabs for Online Data Science
Resolve the captcha to access the links!