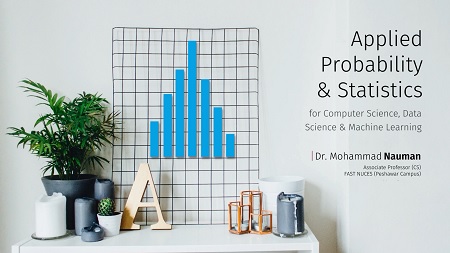
English | MP4 | AVC 1920×1080 | AAC 48KHz 2ch | 6h 35m | 7.88 GB
Real-world, code-oriented learning for programmers to use prob/stats in all of CS, Data Science and Machine Learning
The objective of this course is to give you a solid foundation needed to excel in all areas of computer science—specifically data science and machine learning. The issue is that most of the probability and statistics courses are too theory-oriented. They get tangled in the math without discussing the importance of applications. Applications are always given secondary importance.
In this course, we take a code-oriented approach. We apply all concepts through code. In fact, we skip over all the useless theory that isn’t relevant to computer science. Instead, we focus on the concepts that are more useful for data science, machine learning, and other areas of computer science. For instance, many probability courses skip over Bayesian inference. We will get to this immensely important concept rather quickly and give it due attention as it is widely thought of as the future of analysis!
This way, you get to learn the most important concepts in this subject in the shortest amount of time possible without having to deal with the details of the less relevant topics. Once you have developed an intuition of the important stuff, you can then learn the latest and greatest models even on your own!
Table of Contents
1 Introduction
2 Code Environment Setup and Python Crash Course
3 Getting Started with Code – Feel of Data
4 Foundations Data Types and Representing Data
5 Practical Note – One-Hot Vector Encoding
6 Exploring Data Types in Code
7 Central Tendency Mean Median and Mode
8 Dispersion and Spread in Data Variance Standard Deviation
9 Dispersion Exploration Through Code
10 Introduction to Uncertainty Probability Intuition
11 Simulating Coin Flips for Probability
12 Conditional Probability the Most Important Concept in Stats
13 Applying Conditional Probability – Bayes Rule
14 Application of Bayes Rule in the Real World – Spam Detection
15 Spam Detection – Implementation Issues
16 Rules for Counting Mostly Optional
17 Quantifying Events – Random Variables
18 Two Random Variables – Joint Probabilities
19 Distributions – Rationale and Importance
20 Discrete Distributions Through Code
21 Continuous Distributions with the Help of an Example
22 Continuous Distributions Code
23 Case Study – Sleep Analysis Structure and Code
24 Visualizing Joint Distributions – The Road to ML Success
25 Dependence and Variance of Two Random Variables
26 Expected Values – Decision Making Through Probabilities
27 Entropy – The Most Important Application of Expected Values
28 Applying Entropy – Coding Decision Trees for Machine Learning
29 Foundations of Bayesian Inference
30 Bayesian Inference Code Through PyMC3
Resolve the captcha to access the links!