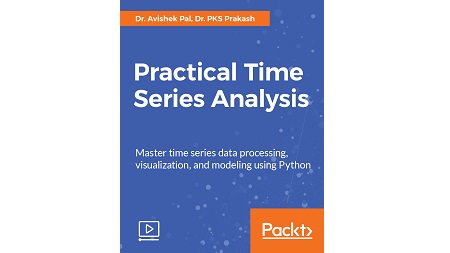
English | MP4 | AVC 1920×1080 | AAC 44KHz 2ch | 2h 25m | 610 MB
Step-by-step guide filled with real-world practical examples
Master Time Series Data Processing, Visualization, and Modeling using Python
Time Series Analysis allows us to analyze data that is generated over a period of time and has sequential interdependencies between the observations. This video describes special mathematical tricks and techniques that are geared towards exploring the internal structures of time series data and generating powerful descriptive and predictive insights. Also, the tutorial is full of real-life time series examples and their analyses using cutting-edge solutions developed in Python. The video starts with a descriptive analysis to create insightful visualizations of internal structures such as trend, seasonality, and autocorrelation. Next, the statistical methods of dealing with autocorrelation and non-stationary time series are described. This is followed by exponential smoothing to produce meaningful insights from noisy time series data. At this point, we shift the focus towards predictive analysis and introduce autoregressive models such as ARMA and ARIMA for time series forecasting. Later, powerful deep learning methods are presented to develop accurate forecasting models for complex time series. All the topics are illustrated with real-life problem scenarios and their solutions by best-practice implementations in Python.
This course takes viewers from basic to advanced time series analysis in a very practical way, and with real-world use cases.
What You Will Learn
- Understand the basic concepts of Time Series Analysis
- Develop an understanding of loading, exploring, and visualizing time-series data
- Explore auto-correlation and master statistical techniques
- Take advantage of exponential smoothing to tackle noise in time series data
- Learn to use auto-regressive models to make predictions using time series data
- Build predictive models on time series data using techniques based on auto-regressive moving averages
Table of Contents
01 The Course Overview
02 Different Types of Data
03 Internal Structures of Time Series
04 Models for Time Series Analysis
05 Autocorrelation and Partial Autocorrelation
06 Advanced Processing and Visualization of Time Series Data
07 Resampling Time Series Data
08 Stationary Processes
09 Time Series Decomposition
10 Introduction to Time Series Smoothing
11 First Order Exponential Smoothing
12 Second Order Exponential Smoothing
13 Modeling Higher-Order Exponential Smoothing
14 Working with AR Models
15 Moving Average Models
16 Building Datasets with ARMA
17 Building Datasets with ARIMA
18 Air Pressure Time Series Forecasting
19 PM 2.mp4
Resolve the captcha to access the links!