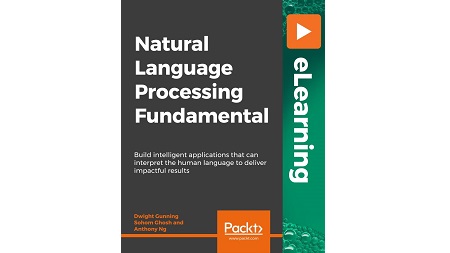
English | MP4 | AVC 1920×1080 | AAC 48KHz 2ch | 6h 03m | 13.8 GB
Use Python and NLTK (Natural Language Toolkit) to build your own text classifiers and solve common NLP problems.
If NLP hasn’t been your forte, Natural Language Processing Fundamentals will make sure you set off to a steady start. This comprehensive guide will show you how to effectively use Python libraries and NLP concepts to solve various problems.
You’ll be introduced to natural language processing and its applications through examples and exercises. This will be followed by an introduction to the initial stages of solving a problem, which includes problem definition, getting text data, and preparing it for modeling. With exposure to concepts like advanced natural language processing algorithms and visualization techniques, you’ll learn how to create applications that can extract information from unstructured data and present it as impactful visuals. Although you will continue to learn NLP-based techniques, the focus will gradually shift to developing useful applications. In these sections, you’ll understand how to apply NLP techniques to answer questions as can be used in chatbots.
By the end of this course, you’ll be able to accomplish a varied range of assignments ranging from identifying the most suitable type of NLP task for solving a problem to using a tool like spacy or gensim for performing sentiment analysis. The course will easily equip you with the knowledge you need to build applications that interpret human language.
Learn
- Obtain, verify, and clean data before transforming it into a correct format for use
- Perform data analysis and machine learning tasks using Python
- Understand the basics of computational linguistics
- Build models for general natural language processing tasks
- Evaluate the performance of a model with the right metrics
- Visualize, quantify, and perform exploratory analysis from any text data
Table of Contents
Introduction to NLP
1 Course Overview
2 Lesson Overview
3 Introduction to NLP
4 Various Steps in NLP – Part I
5 Various Steps in NLP – Part II
6 Lesson Summary
Basic Feature Extraction Methods
7 Lesson Overview
8 Types of Data
9 Cleaning Text Data – Part I
10 Cleaning Text Data – Part II
11 Feature Extraction from Texts
12 Feature Engineering
13 Lesson Summary
Developing a Text Classifier
14 Lesson Overview
15 Machine Learning – Part I
16 Machine Learning – Part II
17 Developing a Text Classifier
18 Building Pipelines for NLP Projects
19 Saving and Loading Models
20 Lesson Summary
Collecting Text Data from the Web
21 Lesson Overview
22 Collecting Data by Scraping Web Pages
23 Requesting Content from Web Pages
24 Dealing with Semi-Structured Data
25 Lesson Summary
Topic Modeling
26 Lesson Overview
27 Topic Discovery
28 Topic Modeling Algorithms
29 Topic Fingerprinting
30 Lesson Summary
Text Summarization and Text Generation
31 Lesson Overview
32 Automated Text Summarization
33 High-Level View of Text Summarization
34 TextRank
35 Summarizing Text Using Different Methods
36 Lesson Summary
Vector Representation
37 Lesson Overview
38 Vector Definition
39 Encoding
40 Word Embeddings and Vectors
41 Lesson Summary
Sentiment Analysis
42 Lesson Overview
43 Sentiment Analysis
44 Sentiment Analysis Tools
45 TextBlob
46 Understanding Data for Sentiment Analysis
47 Training Sentiment Models
48 Lesson Summary
Resolve the captcha to access the links!