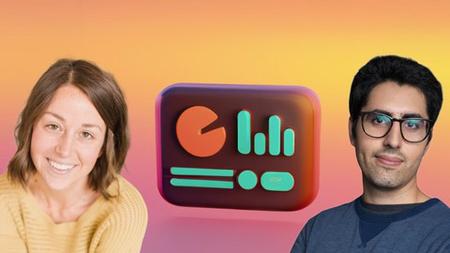
English | MP4 | AVC 1280×720 | AAC 44KHz 2ch | 68 lectures (16h 7m) | 2.91 GB
Learn about probability, statistics, and more using the mathematics that are foundational to the field of data science.
Welcome to the best online course for learning about the Math behind the field of Data Science!
Working together for the first time ever, Krista King and Jose Portilla have combined forces to deliver you a best in class course experience in how to use mathematics to solve real world data science problems. This course has been specifically designed to help you understand the mathematical concepts behind the field of data science, so you can have a first principles level understanding of how to use data effectively in an organization.
Often students entering the field of data science are confused on where to start to learn about the fundamental math behind the concepts. This course was specifically designed to help bridge that gap and provide students a clear, guided path through the complex and interesting world of math used in the field of data science. Designed to balance theory and application, this is the ultimate learning experience for anyone wanting to really understand data science.
Why choose this course?
Combined together, Krista and Jose have taught over 3.2 million students about data science and mathematics and their joint expertise means you’ll be able to get the best and clearest mathematical explanations from Krista with framing about real world data science applications from Jose. At the end of each section is a set of practice problems developed from real-world company situations, where you can directly apply what you know to test your understanding.
What’s covered in this course?
- In this course, we’ll cover:
- Understanding Data Concepts
- Measurements of Dispersion and Central Tendency
- Different ways to visualize data
- Permutations
- Combinatorics
- Bayes’ Theorem
- Random Variables
- Joint Distributions
- Covariance and Correlation
- Probability Mass and Density Functions
- Binomial, Bernoulli, and Poisson Distributions
- Normal Distribution and Z-Scores
- Sampling and Bias
- Central Limit Theorem
- Hypothesis Testing
- Linear Regression
- and much more!
What you’ll learn
- Understand core concepts about data quality and quantity
- Learn about how to measure data with statistics
- Discover how to visualize data with a variety of plot types
- Use combinatorics to calculate permutations and combinations of objects
- Understand the key ideas in using probability to solve problems
- Learn how to use data distributions with real world data
- Discover the powerful insights from the normal distribution
- Use sampling and the central limit theorem
- Understand hypothesis testing on sample groups
- Cover the basics of linear regression
Table of Contents
Introduction
1 Welcome to the Course Important Info in this Lecture
2 Course Overview and Curriculum
Core Data Concepts
3 Introduction to Core Data Concepts
4 Measurements of Central Tendency Mean Median and Mode
5 Measurements of Dispersion Variance and Standard Deviation
6 Quartiles and IQR
Visualizing Data
7 Introduction to Visualizing Data
8 Scatter Plots
9 Line Plots
10 Distribution Plots Histograms
11 Categorical Plots Bar Plots
12 CategoricalDistribution Plots Box and Whisker Plots
13 Other Plot Types Violin Plot KDE Plot
14 Common Plot Pitfalls
Combinatorics
15 Introduction to Combinatorics
16 Factorials
17 Permutations
18 Combinations
19 Combinatorics Practice Problem Set and Answers
Probability
20 Introduction to Probability
21 Probability Law of Large Numbers Experimental vs Expected
22 The Addition Rule Union and Intersection Venn Diagrams
23 Conditional Probability Independent and Dependent
24 Bayes Theorem
25 Discrete Probability
26 Transforming Random Variables
27 Combinations of Random Variables
28 Probability Practice Problem Set and Answers
Joint Distributions
29 Introduction to Joint Distributions
30 Covariance
31 Pearson Correlation Coefficient
32 Joint Distribution Practice Problem Set and Answers
Data Distributions
33 Introduction to Data Distributions
34 Probability Mass Functions
35 Discrete Uniform Distribution Dice Roll
36 Probability Density Functions
37 Continuous Uniform Distribution Voltage
38 Cumulative Distribution Functions
39 Binomial Distribution
40 Bernoulli Distribution
41 Poisson Distribution
42 Data Distributions Practice Problem Set and Answers
The Normal Distribution
43 Introduction to The Normal Distribution
44 Mean Variance and Standard Deviation
45 Normal Distribution
46 Standard Normal Distribution
47 ZScores
48 Normal Distribution Practice Problem Set and Answers
Sampling
49 Introduction to Sampling
50 Sampling and Bias
51 The Central Limit Theorem
52 The Students tDistribution
53 Confidence Interval for the Mean
54 Sampling Practice Problem Set and Answers
Hypothesis Testing
55 Introduction to Hypothesis Testing
56 Inferential Statistics and Hypotheses
57 Significance Level and Type I and II Errors
58 Test Statistics for One and TwoTailed Tests
59 The pValue and Rejecting the Null
60 AB Testing
61 Hypothesis Testing Practice Problem Set and Answers
Regression
62 Introduction to Regression
63 Scatterplots and Regression
64 Correlation Coefficient and the Residual
65 Coefficient of Determination and the RMSE
66 ChiSquare Tests
67 ANOVA
68 Regression Practice Problem Set and Answers
Resolve the captcha to access the links!