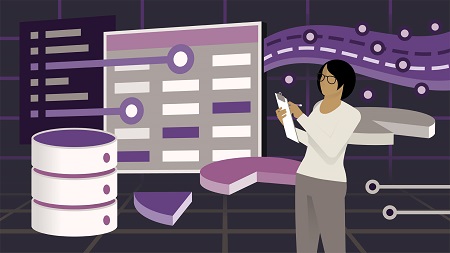
English | MP4 | AVC 1280×720 | AAC 48KHz 2ch | 2h 30m | 324 MB
Many data scientists know how to work with SQL—the industry-standard language for data analysis. But as data sizes grow, you need to know how to do more than simply read and write from a database. This course provides a more sophisticated approach to designing data models and optimizing queries in SQL. Instructor Dan Sullivan begins with the logical and physical design of tables—with particular focus on very large databases—and then presents a deep dive review of indexes, including specialized indexes and when to use them. The next section introduces query optimization and shows how to optimize basic, multi-join, and more complex queries. The course also covers SQL extensions, including user-defined functions and specialized data types. The techniques taught here enable more efficient analysis of large data sets using SQL, statistics, and custom business logic.
Table of Contents
1 Advanced SQL techniques for data science
2 What you should know
3 Rules of normalization
4 Denormalization
5 Partitioning data
6 Materialized views
7 Read replicas
8 Challenge – Design a data model for analytics
9 Solution – Design a data model for analytics
10 B-tree indexes
11 Bitmap indexes
12 Hash indexes
13 GiST and SP-GiST indexes
14 GIN and BRIN indexes
15 Challenge – Choosing an optimal indexing strategy
16 Solution – Choosing an optimal indexing strategy
17 EXPLAIN and ANALYZE commands
18 Generating data with generate sequence
19 Generating time series data
20 Analyzing a query with WHERE clauses and indexes
21 Analyzing a query with a join
22 Challenge – Optimize a query using an explain plan
23 Solution – Optimize a query using an explain plan
24 Extending SQL with user-defined functions
25 SQL query functions
26 Function overloading
27 Function volatility
28 PL Python functions
29 Challenge – Write a user-defined function
30 Solution – Write a user-defined function
31 Federated queries
32 Bloom filters
33 Hstore for key-value pairs
34 JSON for semi-structured data
35 Hierarchical data and ltrees
36 Challenge – Design a table to support unstructured data
37 Solution – Design a table to support unstructured data
38 Next steps
Resolve the captcha to access the links!