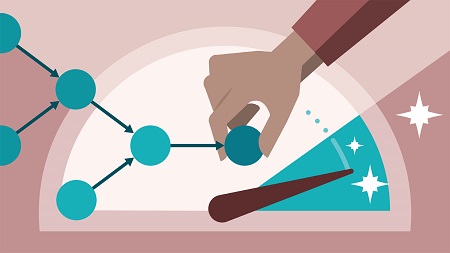
English | MP4 | AVC 1280×720 | AAC 48KHz 2ch | 3h 05m | 350 MB
Discover how to leverage TensorFlow—an open-source software library for numerical computation—to build high-performing machine learning applications. In this course, instructor Matt Scarpino helps to acquaint you with this exciting tool. Here, he explores the process of developing TensorFlow applications and running them on the Google Cloud Machine Learning (ML) Engine.
Matt kicks off the course by discussing TensorFlow development in detail, starting with basic tensor operations and proceeding to graphs, sessions, variables, and training. He also goes over high-level features like datasets, iterators, and estimators. Next, Matt introduces the Google Cloud Platform (GCP) and its capabilities. He shows how to create a GCP project and access it through the Cloud SDK utility. In addition, he covers Google Cloud Storage, which enables developers to upload data that can be accessed in GCP applications. To wrap up, he steps through how to deploy your TensorFlow applications to the ML Engine.
Topics include:
- Creating tensors
- Basic and advanced tensor operations
- Accessing graphs and sessions in code
- Training TensorFlow applications
- Using variables in code
- Using optimizers
- Using TensorBoard in practice
- Accessing data with datasets
- Coding with datasets and iterators
- Machine learning with estimators
- Deploying estimators to the Machine Learning Engine
Table of Contents
Introduction
1 Welcome
2 What you should know
3 Using the exercise files
Introducing TensorFlow
4 Overview and installation
5 Getting started
6 Running a simple application
Fundamentals of TensorFlow Development
7 Creating tensors
8 Basic tensor operations
9 Advanced tensor operations
10 Understanding graphs and sessions
11 Accessing graphs and sessions in code
Training TensorFlow Applications
12 Variables and logging
13 Using variables in code
14 Using optimizers
15 Simple optimizer example
16 Batches and placeholders
17 Linear regression in code – Part 1
18 Linear regression in code – Part 2
19 TensorBoard
20 Using TensorBoard in practice
Accessing Data with Datasets
21 Datasets and iterators
22 Coding with datasets and iterators
23 Dataset operations
24 Creating datasets from files
25 Introducing MNIST images
26 Reading MNIST data in code
Machine Learning with Estimators
27 Understanding estimators
28 Describing data with feature columns
29 Coding a simple estimator – Part 1
30 Coding a simple estimator – Part 2
31 Estimators and neural networks
32 Coding a DNN estimator – Part 1
33 Coding a DNN estimator – Part 2
34 Automating estimator operation
35 Estimator automation in practice
Deploying Estimators to the Machine Learning Engine
36 Creating a GCP project
37 Installing the Cloud SDK
38 Introduction to Google Cloud Storage
39 Accessing Cloud Storage in practice
40 Machine Learning Engine
41 Deploying jobs to ML Engine
Conclusion
42 Next steps
Resolve the captcha to access the links!